Online Seminar: Yihui Quek, Stanford University, CA, USA
A survey of quantum algorithms for statistics and a robust quantum minimum-finding algorithm.
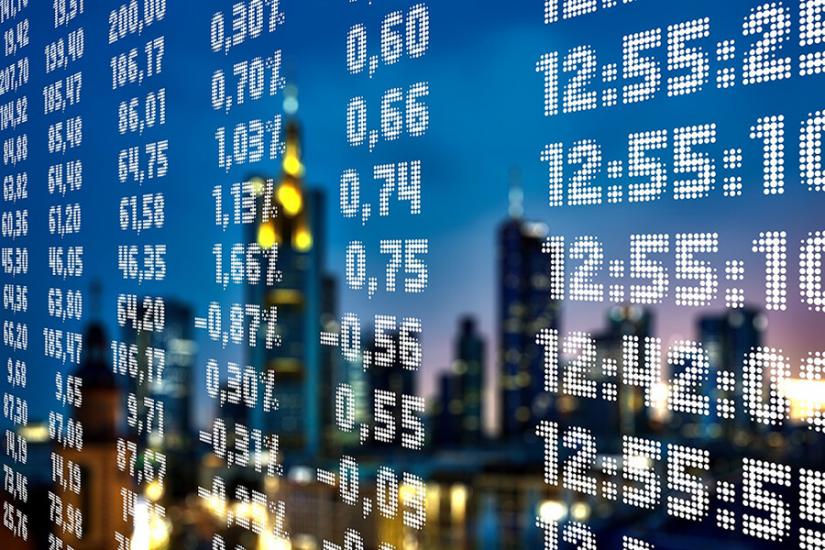
Image: Gerd Altmann/Pixabay
Robust Quantum Minimum Finding
SPEAKER: Yihui Quek
AFFILIATION: Stanford University, California, USA
HOSTED BY: A/Prof Chris Ferrie
ABSTRACT:
Given access to samples from an experiment or population, what properties can we infer about the underlying probability distribution that generated those samples? This question underpins the field of distribution testing, which is fast gaining traction classically due to the generation of ever-increasing amounts of data, over ever-expanding domain sizes. Can quantum computers provide speedups (reductions in sample/query complexity) for these problems?
I will start my talk with a brief survey of the landscape of quantum algorithms for distribution testing and statistical estimation. For the rest of the talk, I will focus on the specific problem of hypothesis selection with N hypotheses. In fact, I will describe a more general quantum algorithm for minimum-finding, for which this is an application: that is, find the minimum of a well-ordered list of length N when the pairwise comparator between the elements is imprecise, or noisy in the following sense: given two elements to compare, if the values of the elements differ by at least α, then the comparison will be made correctly; if the values of the elements are closer than α, the outcome of the comparison is not subject to any guarantees. We show that the quadratic speedup of the 1996 algorithm of Durr and Hoyer is preserved even in such a setting. Even beyond distribution testing, we expect the resulting ‘robust’ quantum minimum-finding algorithm to have further applications in fields ranging from optimisation to finance.